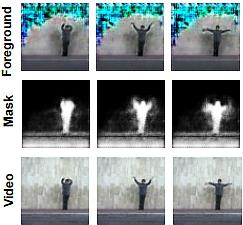
Unconditional Video Generation by efficient decomposition of video features
Date11th Feb 2022
Time04:00 PM
Venue Online event - meet.google.com/byk-gaok-res
PAST EVENT
Details
Generative tasks are widely used to create unseen data by learning the training data distribution. Recent works on video generation focus on creating unseen videos using latent vectors. Video generation is a challenging problem due to the existence of temporal dimension in the data. Also, generated videos may have enormous possible variations, making the task quite complex. One can reduce the complexity of representing a video by decomposing it into various components, like foreground, background, motion, appearance, objects, etc. Many existing methods follow a similar strategy but fail to generate realistic videos, especially for complex datasets.
In general, humans perceive a video in its three components: background, foreground, and motion of the foreground. Inspired by this, we also decomposed the task of video generation into three sub-tasks using a three-branch GAN-based architecture, where two branches model the foreground and background information, whereas the third branch models the temporal information without any supervision. To generate a realistic video, one should focus on two key attributes of a video; temporal coherency between frames and spatial coherence within a frame. To retain the temporal coherency, we introduce a shuffling loss strategy, which guides the network to differentiate between in-order videos and out-of-order (shuffled frame-wise over time) videos. Also, to encourage spatial coherency, we proposed to use a feature-level masking layer, which helps the network for better foreground-background decomposition. We show that our proposed model provides enhanced performance over a few existing recent SOA methods, when verified using benchmark datasets: Shapes, Weizmann Action, UCF101.
Speakers
Arti Keshari
Computer Science and Engg