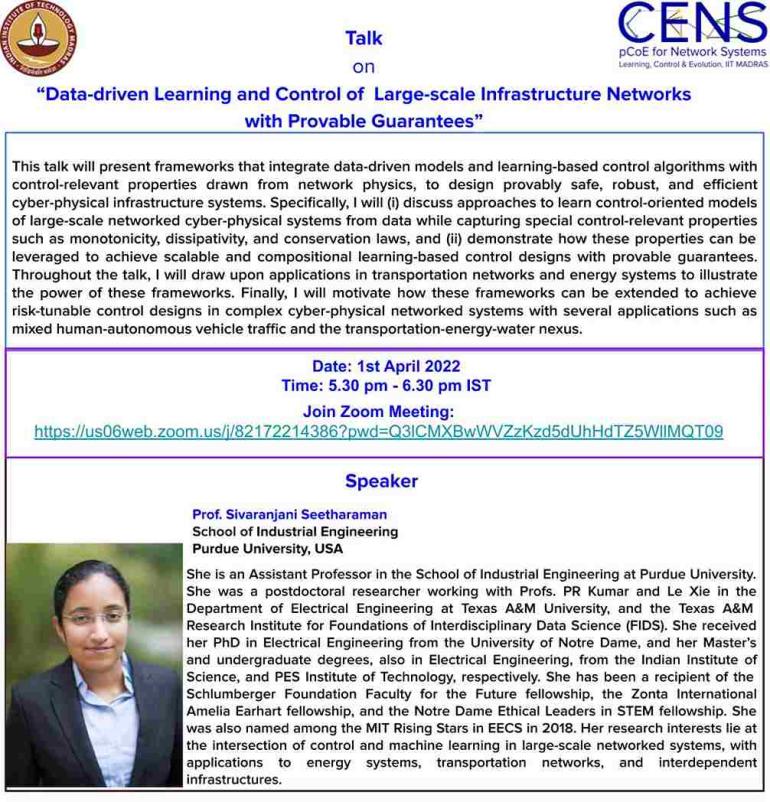
Data-driven learning and control of large-scale infrastructure networks with provable guarantees
Date1st Apr 2022
Time05:30 PM
Venue https://us06web.zoom.us/j/82172214386?pwd=Q3lCMXBwWVZzKzd5dUhHdTZ5WllMQT09
PAST EVENT
Details
Disruptive technological advances in computing, sensing, and communication technologies over the last decade have opened up opportunities for smart cities where large-scale cyber-physical infrastructure networks like energy, traffic, gas, and water can be efficiently monitored, controlled and managed in real-time. Further, the widespread integration of intelligent agents like autonomous vehicles into these traditionally human infrastructures has introduced both opportunities and challenges in the control of these systems. Traditional control techniques do not scale well to such large-scale networks due to complexities arising from the network size, granularity of real-time measurement data, and multi-layered dynamical interactions between the physical networks, computing, communication, and human participants. On the other hand, purely data-driven and learning-based approaches to operating these networks do not provide guarantees on stability, safety, and robustness that are crucial in such safety-critical systems. This talk will present frameworks that integrate data-driven models and learning-based control algorithms with control-relevant properties drawn from network physics, to design provably safe, robust, and efficient cyber-physical infrastructure systems. Specifically, I will (i) discuss approaches to learn control-oriented models of large-scale networked cyber-physical systems from data while capturing special control-relevant properties such as monotonicity, dissipativity, and conservation laws, and (ii) demonstrate how these properties can be leveraged to achieve scalable and compositional learning-based control designs with provable guarantees. Throughout the talk, I will draw upon applications in transportation networks and energy systems to illustrate the power of these frameworks. Finally, I will motivate how these frameworks can be extended to achieve risk-tunable control designs in complex cyber-physical networked systems with several applications such as mixed human-autonomous vehicle traffic and the transportation-energy-water nexus.
Speakers
Prof. Sivaranjani Seetharaman, School of Industrial Engineering, Purdue University, USA
BT/CENS