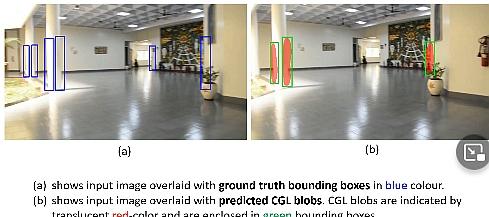
Covert Geo-Location (CGL) Detection using Deep Learning Techniques
Date25th Feb 2022
Time03:30 PM
Venue Online event - google meet
PAST EVENT
Details
The primary focus of the computer vision community has been on the understanding of visual scenes. To this end, many datasets and tasks have been proposed over the years to build AI systems that can perform specific scene understanding tasks. However, most vision-related tasks demand knowledge about only the objects present in the scene and the relationships between them. Other non-object image regions such as hideouts, turns, and other obscured regions also contain crucial information, for specific surveillance tasks. In this work, we propose an intelligent visual aid for identification of such non-object locations (specifically, potential hideouts or Covert Geo-Locations) in an image of an indoor scene, which either have the potential to cause an imminent threat or appear as the target zone needing access for further investigation to identify any occluded objects. We have termed our task as Covert Geo-Location (CGL) Detection. Occluding items (such as furniture, pillar, curtains) themselves are not specifically the targets here in the proposed task, but regions for access to the zones occluded by them are so. Thus, the goal in the novel task of CGL detection is to identify certain regions around sub-segments of outer boundaries of occluding items in an image, which need to be accessed for further investigation. CGL detection finds applications in military counter-insurgency operations and general surveillance with or without path planning for an exploratory robot.
CGL detection requires context-aware detection and understanding of the complex 3D spatial relationships between boundaries of occluding items and their surroundings. Depth information is also very crucial for the detection of CGLs. Thus we have developed an approach that can effectively extract RGB-based features as well as relevant depth features, using only a single RGB image as input. A novel DL-based technique has been proposed which uses an auxiliary decoder block named Depth-aware Feature Learning Block (DFLB) to steer the feature extractor towards extraction of relevant depth features (along with other necessary features). Additionally, as the proposed dataset (1.5K CGL annotated images) is relatively small, we have leveraged two novel self-supervised feature-level loss functions namely, Geometric Transformation Equivariance (GTE) loss and Intraclass Variance reduction (IVR) loss to enforce additional constraints on the model, so that it recognizes key aspects of CGLs which are helpful for their detection. Experimental evaluations, performed on our proposed novel CGL Dataset, demonstrate a significant increase in performance over the existing object detection and segmentation models (when adapted and trained from scratch for CGL Detection), serving as a testimony to the superiority of our proposed approach. The future task involves the use of transformers and graph neural networks for enhancement in the performance of CGL detection.
An online live demo of CGL detection will be illustrated at the end of talk.
Speakers
Mr. Binoy Sarkar (CS19S024)
Computer Science and Engg